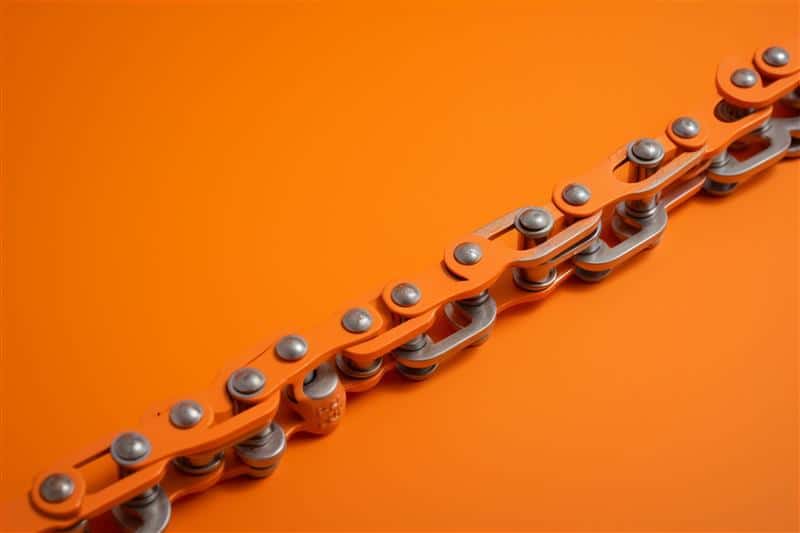
Artificial Intelligence (AI) continues to reshape industries and daily life, yet many still find its inner workings — especially how it generates responses — mysterious. At its core, AI prompting involves feeding input data to models, which then generate outputs based on the learned patterns. However, without transparency, the reasoning behind these outputs often feels like a “black box”, leaving users unsure of how AI reaches its conclusions and whether they can trust its recommendations.
Striking a balance between innovative, free-flowing responses and precise, traceable conclusions remains a key challenge in AI development. In this blog, Be Informed has collaborated with Knowit, our partner specialized in optimizing and automating business processes with tailored solutions, to bring you clarity on what Chain-of-Thought prompting is and how it can be helpful to businesses working with AI.
Chain-of-Thought Prompting: Creative vs Accurate
What is Chain-of-Thought Prompting?
Chain-of-Thought (CoT) prompting is a method that encourages AI to “think out loud,” describing its reasoning process step-by-step. This approach ensures that the final output reflects a logical reasoning path, enhancing reliability. In recent years, CoT prompting has gained traction as a solution to the unpredictable variations in AI’s responses and its limitations in handling complex, multi-domain problems.
Structured vs Unstructured CoT Prompting
Structured CoT prompting focuses on a systematic and traceable approach to problem-solving. By breaking down tasks into smaller, logical steps, structured CoT ensures that the AI’s reasoning process is transparent and aligned with specific goals. This method is particularly valuable when dealing with high-stakes scenarios, such as legal compliance or financial modeling, where accuracy and consistency are prioritized.
Unstructured CoT, on the other hand, allows for more creativity. It enables AI to explore diverse perspectives and generate imaginative responses without rigid constraints. While this approach is useful for brainstorming or generating artistic content, it often lacks the traceability and reliability needed for critical decision-making.
Benefits of Each Approach
- Structured CoT: Enhances accuracy, transparency, and user trust. It ensures that AI outputs are explainable and can be aligned with ethical or legal standards.
- Unstructured CoT: Fosters creativity and innovation, making it ideal for exploratory tasks and creative industries.
The choice between these approaches depends on the context in which AI is used. However, as AI becomes integral to decision-making processes, the importance of structured CoT is growing.
The Importance of Traceability with AI
In critical situations, understanding how AI arrives at its conclusions is not just a technical necessity — it’s a legal and ethical imperative.
Legal Implications
In legal and regulatory environments, traceability ensures that AI decisions are consistent, unbiased, and explainable. For instance, when AI systems interpret laws or provide recommendations in legal cases, stakeholders need to verify that these outputs adhere to relevant statutes and ethical guidelines. A lack of traceability can lead to significant consequences, from misinterpretation of laws to biased rulings.
Building User Trust
Users are more likely to trust AI systems that provide transparent reasoning. Traceable outputs allow users to follow the decision-making process, reducing the risk of blind trust in potentially flawed or biased AI outputs. This transparency is crucial for fostering a culture of responsible AI usage.
RegTech Applications
In regulatory technology (RegTech), structured chain-of-thought prompting enhances traceability and compliance. Since working with AI becomes integral to business processes.
Nowadays, governing its use is growing in importance, especially within strictly regulated industries. By incorporating clear classifications, assessments, and diagnostics into its reasoning process, structured CoT helps organizations ensure that AI systems remain transparent, accurate, and aligned with regulatory frameworks. This approach reduces compliance risks and multiplies trust in AI-driven decisions.
A Real-Life Example: Child Custody Case
Senior Data Scientist from Knowit, Tim Bervoets, has explained how a rigorously structured Chain-of-Thought approach can make a huge difference when working with family law:
“Imagine a child custody case involving accusations of emotional manipulation, conflicting evidence on the child’s preferences, and potential parental alienation. Deciding what’s in the best interest of the child requires considering legal standards, psychological evaluations, and ethical considerations. This is complex and multi-dimensional, so unstructured Chain-of-Thought prompting might not be enough”.
He continues:
“Some of the reasoning challenges that make cases like this difficult for AI to process are:
- Admissibility of Evidence: Evaluating if evidence on the child’s preferences and manipulation is relevant and admissible.
- Parent-Child Dynamics: Examining each parent’s relationship with the child for signs of alienation.
- Child’s Best Interests: Identifying the custody arrangement that supports the child’s well-being.
- Allegation Credibility: Assessing the reliability of each parent’s claims for bias or inaccuracies.
- Child’s Preferences: Determining if the child’s choices are genuine or influenced by manipulation.
Parental Alienation: Investigating if a parent’s actions qualify as alienation. - Emotional Manipulation: Measuring the presence and impact of manipulation on the child.
- Long-Term Impact: Predicting how custody decisions will affect the child’s future well-being.
- Risk of Recurrence: Estimating the likelihood of alienation or manipulation continuing”.
How can Structured CoT help?
In his LinkedIn article, Bervoets shows how a formally structured CoT approach could revolutionize such cases by providing a systematic and transparent reasoning process. For example:
1: Restate the problem and success criteria clearly, outlining the legal and ethical frameworks involved.
2: Define assumptions and gather relevant data (e.g., legal precedents, psychological studies).
3: Apply systematic reasoning to weigh evidence and align recommendations with the child’s best interests.
4: Present a transparent explanation of the conclusion, allowing stakeholders to understand and validate the reasoning process.
Such an approach not only enhances decision-making and provides a reasoning trace, but also builds trust among all parties involved.
So, Which One Is It: Structured or Unstructured CoT?
As businesses and industries increasingly integrate Artificial Intelligence into their operations, understanding and leveraging Chain-of-Thought prompting is crucial. Structured CoT prompting provides the transparency, accuracy, and traceability required for AI to become a trustworthy and reliable partner in decision-making, while unstructured CoT remains a valuable tool for fostering creativity and innovation but lacks the ability to handle complex, high-stakes scenarios effectively.
By embracing these methods, organizations can balance creativity with accountability, empowering AI to not only generate innovative ideas but also support critical decisions with confidence and precision.